- Home
- Teaching & Learning
- Specialist Sector
- What AI tells us about communicating with children
What AI tells us about communicating with children
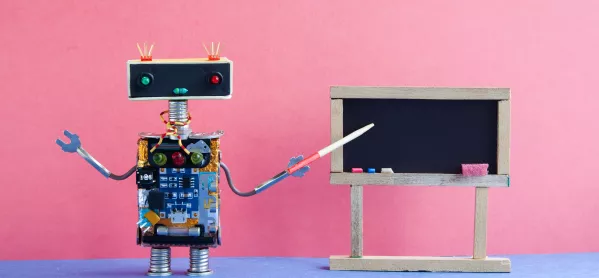
How many interactions do you have with the pupils in your care throughout the day? A seminal study estimated that the number of words, nods, smiles and other gestures that teachers typically use to communicate with their students in any given hour in a mainstream classroom is in the range of 200 to 300 (Jackson, 1969).
Research shows that the way teachers communicate with their pupils makes a huge difference to how well they learn (Stronge, 2011). We know that this is even more important for children who are neurodiverse. But which communication techniques work the best?
Our research, published this September, helps to answer this question. We, along with our co-author Xiao Qu, used artificial intelligence to identify effective communication strategies for children with autism. It might sound complicated, but it wasn’t really: we recorded interactions between teachers and autistic students in the classroom, and then used AI to identify which teacher communications worked and which did not.
More:
- Three things schools need from the SEND review
- Is tech the answer to creating more confident readers?
- 5 ways senior leaders can better support Sendcos
We spent 20 half-days in a special school in London that had criteria of autism spectrum condition for admission. There, we observed which strategies three teachers used to communicate with seven children - words, visual images, objects, gestures or physical prompts - and we observed how well the pupils responded. We logged a total of 5,500 interactions.
How did this work in practice? Well, a teacher was doing a yoga lesson with the whole class, for example, and they demonstrated a particular yoga move by gesturing while also verbally explaining how to do it, and asked the students to do the same. If students responded by doing the move, it was recorded as a positive response. If they didn’t, it was recorded as a non-positive response.
Apart from recording the communication strategies deployed by the teacher and the corresponding outcome (student response), we also captured other data including the teaching objective (academic, social or pedagogic), the general context (eg, whole-class versus working with groups or an individual child), the emotional state of the student (positive, negative or neutral), and basic student attributes (eg, age and academic level). Based on a subset of all recorded interactions, we created a machine-learning model.
The model uses the teacher communication strategy and the other collected data items to predict student response. We then measured the accuracy of the model in predicting student response on a separate subset of interactions that we did not use for training. The model correctly predicted what the outcome would be 70 per cent of the time.
More specifically, the model provides an estimate of the probability of a positive student response. Now, in a given situation - for example, a child of a particular age and academic profile in a particular teaching scenario - we can use our model to evaluate different teacher communication strategies by obtaining a probability for a positive student response for each one of them.
In that way, we can select the communication strategy that maximises the probability of a positive student response. This is the AI-driven suggestion that the model presents to a teacher.
We also conducted a statistical analysis, looking at the longer-term effects of specific teacher communication strategies. We concluded that visual prompts (the use of a picture or an object) have a positive effect that persists over time, but more intrusive strategies (eg, a physical prompt) may immediately enforce the sought outcome, but over time the student response can be negatively affected.
Communication with autistic children: why this research matters
Why does all of this matter? Well, we know that children and young people with autism tend to communicate in different ways to neurotypical people. The extent of neurodiversity and how it might be expressed can vary considerably from one person to another, which is why we talk about autism as being a spectrum.
Autistic people can have particular strengths, such as the ability to focus very effectively on detail (Lorenz and Heintiz, 2004). They can also, however, find social communication a challenge. They can struggle to make sense of other people’s intentions or perspectives and vice versa.
Managing and expressing emotions can be tricky too, which in turn can make navigating typical social situations difficult. Some children on the spectrum might also take longer to start speaking or to understand how to use certain forms of speech, for example, how to ask questions (Onur, 2011). They might also have specific preferences, such as responding more positively when a teacher uses pictures or real objects as aids rather than other forms of communication.
The range of challenges that autistic children face in communication exacerbates the difficulty of deriving effective teaching strategies. How can teachers help all their pupils understand classroom routines or cooperate with other children? Traditionally, research in autism education has relied on what teachers think are the most effective ways to communicate.
However, research shows that there are limitations to the extent to which teachers can figure out what is the best strategy, even after they have worked with an autistic child for a considerable length of time.
If methods such as the one presented in our study could help teachers choose the right communication strategy for a certain situation, then this could make a difference in how children progress socially as well as academically.
However, we are not there yet - 70 per cent predictability is good, but it could be better. Essential next steps include the development of more accurate and inclusive machine-learning solutions with the addition of more data, and their extensive assessment in practical settings.
Vasileios Lampos is an associate professor in the Department of Computer Science at University College London, and Dr Joseph Mintz is an associate professor in education in the Department of Learning and Leadership at UCL’s Institute of Education
You need a Tes subscription to read this article
Subscribe now to read this article and get other subscriber-only content:
- Unlimited access to all Tes magazine content
- Exclusive subscriber-only stories
- Award-winning email newsletters
Already a subscriber? Log in
You need a subscription to read this article
Subscribe now to read this article and get other subscriber-only content, including:
- Unlimited access to all Tes magazine content
- Exclusive subscriber-only stories
- Award-winning email newsletters
topics in this article