Look beyond the label
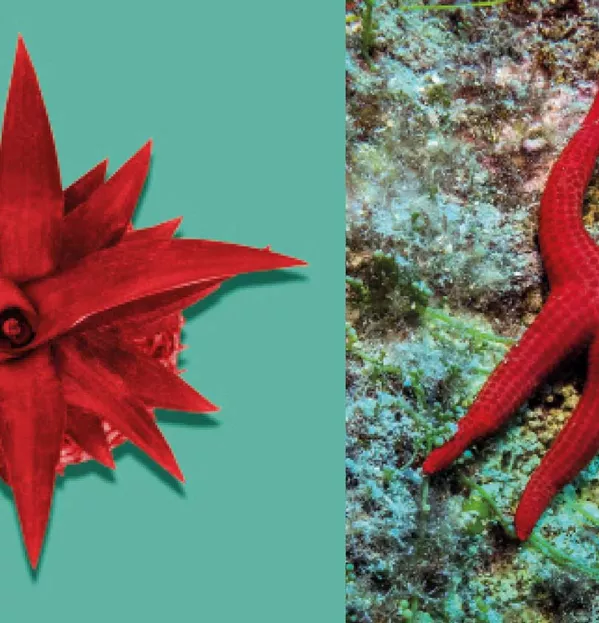
Alfie is eight years old and already has a reputation in his primary school. His Reception, Year 1 and Year 2 teachers found his behaviour difficult to manage: he wouldn’t sit still, he often disrupted other children and he found it hard to pay attention.
Academically, he struggled, too: he was making slower progress in reading than many of his peers.
So, when he started Year 3, his new teacher, Sarah, decided something had to change. She discussed Alfie with his previous teachers, the special educational needs and disabilities coordinator (Sendco) and his parents. Sarah’s meeting with his family proved pivotal: they told her Alfie’s behaviour at home was the same as in the classroom. Sarah suggested a chat with a GP might be helpful.
Alfie was referred by his GP to child and adolescent mental health services and was diagnosed with attention deficit hyperactivity disorder (ADHD).
At last, the school and Alfie’s parents had an answer, and the correct support would follow, right? Not necessarily.
Up to 15 per cent of the school population are recognised as having special educational needs (SEN). But what do we actually know about why so many children find learning difficult? We’ve been addressing this question through our work at the Centre for Attention Learning and Memory (Calm), at the MRC Cognition and Brain Sciences Unit, University of Cambridge. And a recent study we published in the journal Developmental Science found evidence for something that many who work in special schools have suspected all along: we rely far too heavily on diagnosis to support those with SEN, and that diagnosis rarely relates to the underlying reasons why a child is struggling.
What follows is a version of this recent paper, which has been exclusively tailored for Tes’ audience.
Part 1
Following the diagnosis
It is not just Alfie who has a diagnosis of ADHD in Sarah’s class. She has two other pupils with the same diagnosis, and all three take methylphenidate (Ritalin). The three children, however, are very different in their behaviour and attainment in school.
Alfie, as we know, is struggling with both behaviour and attainment. One of the other children is making good progress with few behavioural issues. The third child is struggling academically but her behaviour is not as disruptive as Alfie’s - she can often be found daydreaming or looking out of the window rather than paying attention to the task at hand.
Then there are the other children in Sarah’s class with different challenges. There is Jessie, aged 7. Her reading level is that of a child almost three years younger. Following discussions between the Sendco and Jessie’s family, the school organises an assessment with an educational psychologist. The psychologist’s report states that Jessie has dyslexia; it provides Sarah with some teaching strategies and materials that she can use in the classroom with Jessie. Sarah has a teaching assistant who spends half a day per week providing additional support to Jessie in the classroom, guided by the materials provided by the educational psychologist.
And then there is Ella, who is also 7. Sarah has noticed that her reading is poor and that it is affecting other aspects of her learning. Ella does not have a diagnosis, but Sarah has liaised with the Sendco, who has initiated “school action” in line with the SEND code of practice. She is waiting for the first meeting to decide whether Ella has SEN and what action might follow to support her needs. At the moment, Ella receives no additional support at school.
Despite all four of the five children displaying similarly low levels of reading, Sarah is being guided by the labels given to the children and recommended approaches for intervention (medication for ADHD; one-to-one teaching-assistant support for dyslexia; school action for SEND).
This should work. Their reading skills should improve. Shouldn’t they?
Part 2
What do learning difficulties really look like?
Learning difficulties and associated developmental problems do not fall into tightly defined categories. Symptoms often co-occur across diagnoses, and two children with the same diagnosis can behave in very different ways.
For example, it is not uncommon for a child with ADHD and another with dyslexia to both have problems sustaining attention during challenging tasks. Similarly, no two children with ADHD will behave the same: some are more hyperactive and defiant while others are less disruptive and more inattentive. Stepping back from diagnoses, we also see that classroom learning difficulties rarely occur in isolation. There are high rates of comorbidity between reading, maths and language problems, with some estimates reaching up to 50 per cent. This is not to mention strong overlaps with mental health; according to the Royal College of Psychiatrists, up to 40 per cent of people with learning problems experience mental health difficulties.
In practice, many teachers and educational professionals recognise the complex needs of children who are struggling at school. Some leading special schools have stressed the need to move away from labels and instead focus on the individual child and their needs, using diagnostics as supplementary information when needed (bit.ly/DitchLabels). A similar move has been advocated for many years in developmental science.
But to date, very few studies have adopted such an approach.
Part 3
Studying struggling learners: make no assumptions
The use of phonics to teach reading is a great example of how scientific evidence has fed through to everyday classroom practice. But our understanding of learning-related difficulties, and how to support them, is dominated by studies of children with either very specific profiles of learning problems (such as reading difficulties in the absence of maths problems or low IQ), or of children with particular diagnoses in the absence of co-occurring problems or diagnoses.
This approach makes lots of assumptions about struggling learners that practitioners know may not be valid; learning difficulties are rarely specific, behavioural problems rarely occur in isolation and most children who struggle will never receive any diagnosis.
A similar issue was recognised in the field of psychiatric disorders and adult mental health a few years ago. Here, scientists realised that the majority of studies failed to capture high levels of co-occurrence between anxiety and depression, differences in symptoms for individuals diagnosed with the same condition, or similarities in symptoms across diagnoses.
This prompted the adoption of what has since become known as a “transdiagnostic approach” - that is, identifying the causes of particular groups of symptoms, irrespective of diagnosis.
This approach makes no assumptions about why someone might be struggling based on previous information, such as a diagnostic label. This is what we need more of when it comes to struggling learners.
Part 4
The study: what we did and why
We wanted to conduct a truly “transdiagnostic” study of children who struggle in school. Our main challenge was collecting data from a large sample of children who were recognised as having learning-related problems, irrespective of any labels they may or may not have had.
The key to our success would be whether we could recruit a deliberately broad sample of struggling learners.
Our colleague Susan Gathercole pitched the idea of opening a “research clinic”: we would take referrals from practitioners for children who appeared to be struggling. It was an ambitious idea but it proved pivotal.
We asked health and educational professionals (Sendcos, speech and language therapists, specialist teachers, psychologists, psychiatrists and paediatricians) to refer children aged 5-18 to the Calm research clinic. Formal diagnoses were not required and no exclusions were made on the basis of comorbid psychiatric, psychological or physical health conditions.
We accepted children on to the study with none, one or multiple diagnoses. As long as someone with expertise in recognising a cognitive-related learning problem said they were struggling, we took them in.
Initially, we did not know whether anyone would refer children to us, but we should not have worried. To date, we have seen nearly 800 children. Here, we are telling you about the results of a particular analysis we ran on the first 550 children seen in the clinic.
All children completed a broad set of assessments of cognitive abilities known to be impaired in children with learning difficulties. These included tests of vocabulary, sound processing (phonological processing), memory, problem-solving (executive function and IQ) and attention. They also completed assessments of maths and literacy skills.
Children were offered an optional MRI brain scan, and parents/carers completed multiple questionnaires about family history and the child’s behaviour, mental health and communication skills.
The breadth of the recruitment criteria, the scale of the study and the multiple levels of assessment make this study a unique resource for understanding why some children struggle at school. There are so many interesting questions that you can ask of a dataset like this - and, within Calm, many researchers are working to make the most of this resource. The analysis approach we used in this study was machine learning. Our aim was to understand more about the sample of struggling learners we had recruited and, in particular, how their cognitive difficulties related to the particular reasons for referral, their diagnoses and their learning profiles.
It sounds fancy but really it isn’t. Machine learning is a branch of computer science related to artificial intelligence that we use every day without knowing it. Each time you type a word into an internet search engine, a machine-learning algorithm learns about your interests and preferences. It is trying to learn the structure of the input you provide - that is, it is trying to learn the consistent patterns. The machine learning creates a model of your preferences and interests, based on your search history, which is then used to suggest things you might like.
We used a class of machine learning called an “artificial neural network”. These have been popular in lots of other areas of science; they’ve been used to learn about weather systems, economic patterns, seismic activity and ocean currents. When you don’t know the structure of your data - like a new search engine that has no prior knowledge of its user - machine learning is a powerful way of learning that structure.
So, having collected information from hundreds of struggling learners, we used the algorithm to learn about the consistent patterns in the data. We fed the artificial neural network information about children’s vocabulary, listening skills, various types of memory and reasoning ability.
What would the algorithm learn about the structure of this input?
Part 5
Can it distinguish between apples and oranges?
Imagine for a moment you knew nothing about fruit and wanted to use machine learning to help you. First, you would have to define some sensible ways in which fruit could differ from one another - their diameter, colour, acidity, seed size, juiciness, and so on. Second, you would need to measure all these attributes in every fruit. Third, you would have to introduce these values to the machine learning. As the fruits are introduced, the algorithm gradually sorts them according to these attributes.
Our version of machine learning represents what it has learnt as a map. The more similar the fruits, the closer they sit on the map. For example, lemons and limes will sit alongside each other because they are very similar.
By contrast, bananas would be represented somewhere else on the map because they have very little in common with either lemons or limes.
So, what did our map look like in this study? We learnt that many children had consistently poor scores on all our assessments; some children struggled with tasks that involved working memory, some children were really poor at any tasks that relied on verbal skills and (to our surprise) a sizeable portion of the sample had no cognitive difficulties at all. We predicted that children with, say, ADHD would sit together on the map. After all, they have the same diagnosis so they must be very similar to each other. But that was not the case.
For example, a child with an ADHD diagnosis was equally likely to sit anywhere within the map, indicating that the diagnosis did not correspond to their cognitive profile. In fact, the same was true of other diagnoses that were represented within the clinic, such as dyslexia or autism spectrum disorders.
So, the machine learning mapped the profiles of children who struggle at school. These profiles were strongly linked to their academic performance, language ability and brain structure. But they were not strongly linked to any diagnoses the children came with. Now, this is not to say that these diagnoses are not important.
A diagnosis is a landmark moment for children and families, and should lever additional support for that child. But when providing practical support, it is important to keep in mind that diagnosis does not correspond closely with the child’s underlying cognitive difficulty.
Another key point from this study is that most struggling learners we saw did not have a diagnosis of any kind: having a diagnostic label was not a good predictor of whether a child would struggle or not, let alone why they might struggle.
The machine learning also taught us something else that we had not expected: children can have similar profiles of learning but for different underlying reasons. In our data, two children in a class could both have moderate learning problems in reading and maths, but with different underlying roots. One might have underlying problems in verbal skills or phonological processing, while the other might be struggling owing to problems with working memory.
This presents a real challenge for practitioners because the two children will present similarly in class but the underlying cognitive origins of those difficulties could be somewhat different.
Part 6
So, what do we do now?
We would recommend the following, based on our findings:
1. Better, easier and cheaper assessments
When we speak at practitioner conferences, a common question that we hear is “where can professionals get their hands on cognitive assessments?” Despite being incredibly simple, most are prohibitively expensive. That needs to change.
2. Better-funded SEN provision
Most schools we worked with could not afford to obtain support from an educational psychologist. Indeed, we suspect that one reason recruitment to our study was so successful was because it provided the only means of professionals getting access to this kind of cognitive data on the children. Tailored help for children requires that they have support from educational psychology services. In our experience, this has become impossible for most schools.
3. Sendco and teacher training
Knowing the ways in which cognitive profiles can differ, and how they correspond to learning, could be really useful information for teachers. For example, many of our struggling learners had working-memory problems. Reducing working-memory demands in the classroom with different tricks and strategies can significantly improve learning outcomes for children who have poor working-memory capacity. Integrating this within teacher and Sendco training would be valuable.
This should present a change in mindset, too. Our study is the first to map cognitive profiles in such a broad group of struggling learners. It clearly demonstrates that diagnoses do not map on to cognitive skills.
These findings underscore the need to understand more about why children are struggling in the classroom rather than simply adopting off-the-shelf interventions for particular labels.
The key message is that children with the same diagnoses do not necessarily have the same underlying cognitive problems. What works for one child with a diagnosis might not work for another child with the same diagnosis. We adopted one method of analysis - machine learning - but many others are available that may provide additional or complementary information.
We believe the challenge ahead is to develop ways to chart profiles across learning, cognition, behaviour, the brain and genes. This way, we will gain richer information linking particular profiles of learning-related problems to (potentially different) underlying causes. As we push our understanding of which mechanisms can give rise to difficulties in learning, we can better target our interventions.
Finally, it is crucial to map changes in cognition and learning over time to identify risk and resilience factors for persistent learning difficulties. We are about to start a five-year follow-up of the children referred to Calm to do just this. Watch this space.
Dr Joni Holmes is head of the Centre for Attention, Learning and Memory at the Medical Research Council’s Cognition and Brain Sciences Unit (MRC CBU), and a fellow of Wolfson College; Dr Duncan Astle is a programme leader at the MRC CBU and a fellow of Robinson College; and Dr Joe Bathelt is a postdoctoral researcher at the MRC CBU, all at the University of Cambridge. The clinic provides free resources for professionals supporting struggling learners (see bit.ly/CambCalm)
Read the original paper
Astle, DE, Bathelt, J, Holmes, J et al (2018) “Remapping the cognitive and neural profiles of children who struggle at school”, Developmental Science, bit.ly/RemapCog
You need a Tes subscription to read this article
Subscribe now to read this article and get other subscriber-only content:
- Unlimited access to all Tes magazine content
- Exclusive subscriber-only stories
- Award-winning email newsletters
Already a subscriber? Log in
You need a subscription to read this article
Subscribe now to read this article and get other subscriber-only content, including:
- Unlimited access to all Tes magazine content
- Exclusive subscriber-only stories
- Award-winning email newsletters